Lingxiao Zhao
Carnegie Mellon University
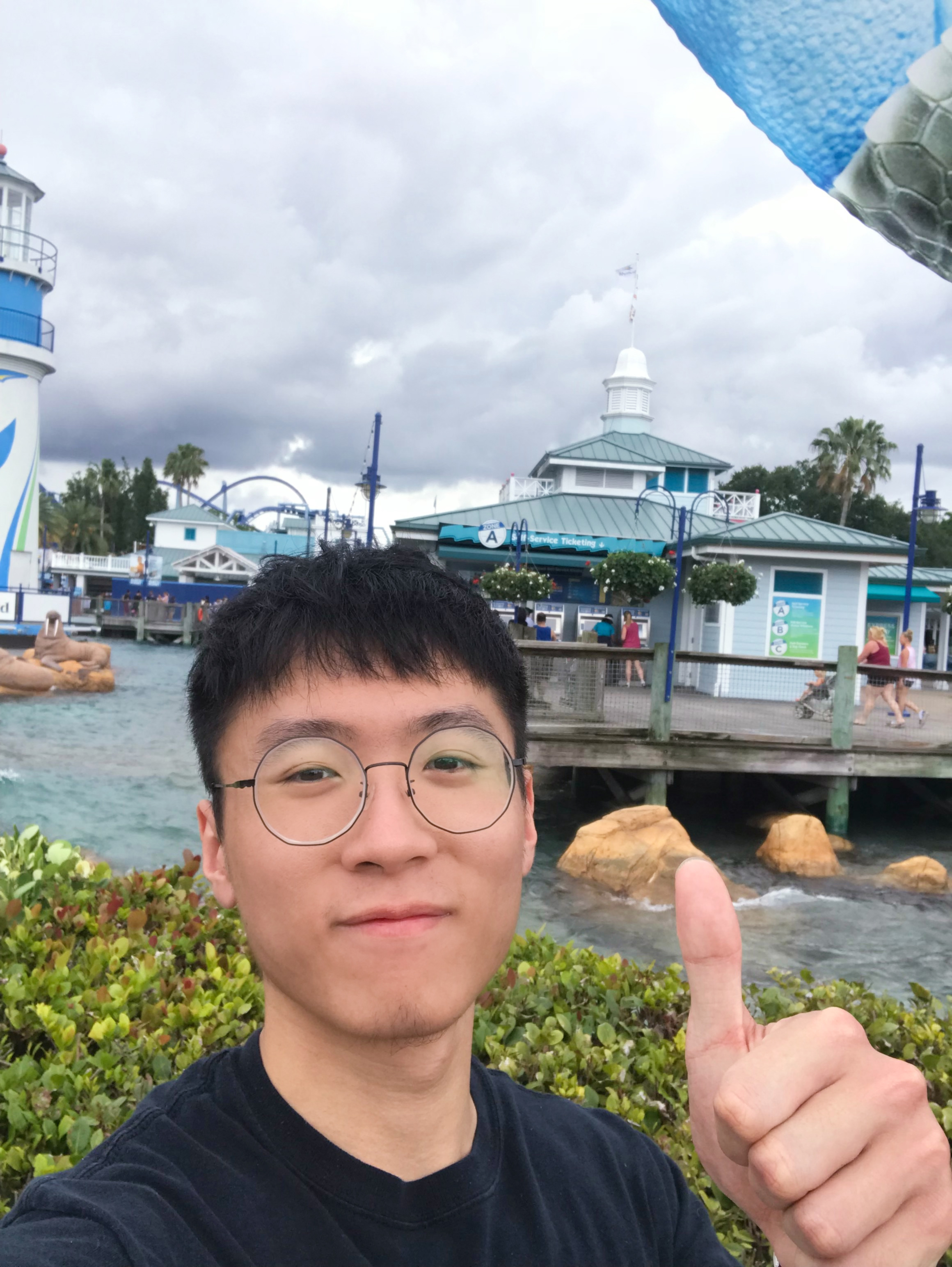
I’m a last year Ph.D. student of Machine Learning Joint Public Policy in Machine Learning Department and Heinz College at Carnegie Mellon University, advised by Prof. Leman Akoglu. My research mainly focuses on developing machine learning algorithms over graph-structured data, and applying designed algorithm to solve real-world problems. In the meantime, I have worked on pretraining LLMs, diffusion based generative models. In future, I’m interested in combining graph models and LLMs to boost LLMs’ reasoning and emergent abilities in different stages, and towards a multimodal generative foundation model.
I finished my Master’s in ECE department at Carnegie Mellon Univrsity, and Bachelor’s (while I was in power system area) in EE department at Xi’an Jiaotong University.
I have a twin brother Lingfei Zhao pursuing his Ph.D. of Physics at Duke University. We enjoy games together :).
Email: my first name at cmu.edu
news
Feb 1, 2024 | Improving and Unifying Discrete- and Continuous-time Discrete Denoising Diffusion and Pard: Permutation-Invariant Autoregressive Diffusion for Graph Generation are now available at arxiv! If you work on discrete/categorical data’s generative model, don’t miss our paper that unifies and improves discrete denoising diffusion. Also, Pard is a the first permutation-invariant graph generative model that combines the strength of diffusion models and autoregressive methods. Pard achieves greatly improved performance on many benchmarks! |
Oct 1, 2022 | A Practical, Progressively-Expressive GNN and Hyperparameter Sensitivity in Deep Outlier Detection: Analysis and a Scalable Hyper-Ensemble Solution are accepted at NeurIPS 2022! |
Jan 24, 2022 | From Stars to Subgraphs: Uplifting Any GNN with Local Structure Awareness and Graph Condensation for Graph Neural Networks are accepted at ICLR 2022! |
Oct 10, 2021 | Patches are all you need? Subgraphs are all you need!! We propose a theoretically proved general framework to boost any GNN’s expressiveness without losing scalability: From Stars to Subgraphs: Uplifting Any GNN with Local Structure Awareness |
Aug 23, 2021 | Our work On Using Classification Datasets to Evaluate Graph Outlier Detection: Peculiar Observations and New Insights is accepted by Big Data Journal! |
Jun 23, 2020 | Our work Connecting GCN and Graph-Regularized PCA is accepted by GRL+ Workshop of ICML 2020! |
Dec 19, 2019 | Our work PairNorm: Tackling Oversmoothing in GNNs is accepted by ICLR 2020! |
Oct 6, 2019 | Start my academic personal website! |